With Eugene Kanevsky, Co-Head of Trading and Execution Services; James Redbourn, Head of Electronic Execution Client Services; and Joanna Wong, Head of Electronic Execution Platform Strategy, CLSA
How would you characterize the current landscape for electronic equity trading in Asia?
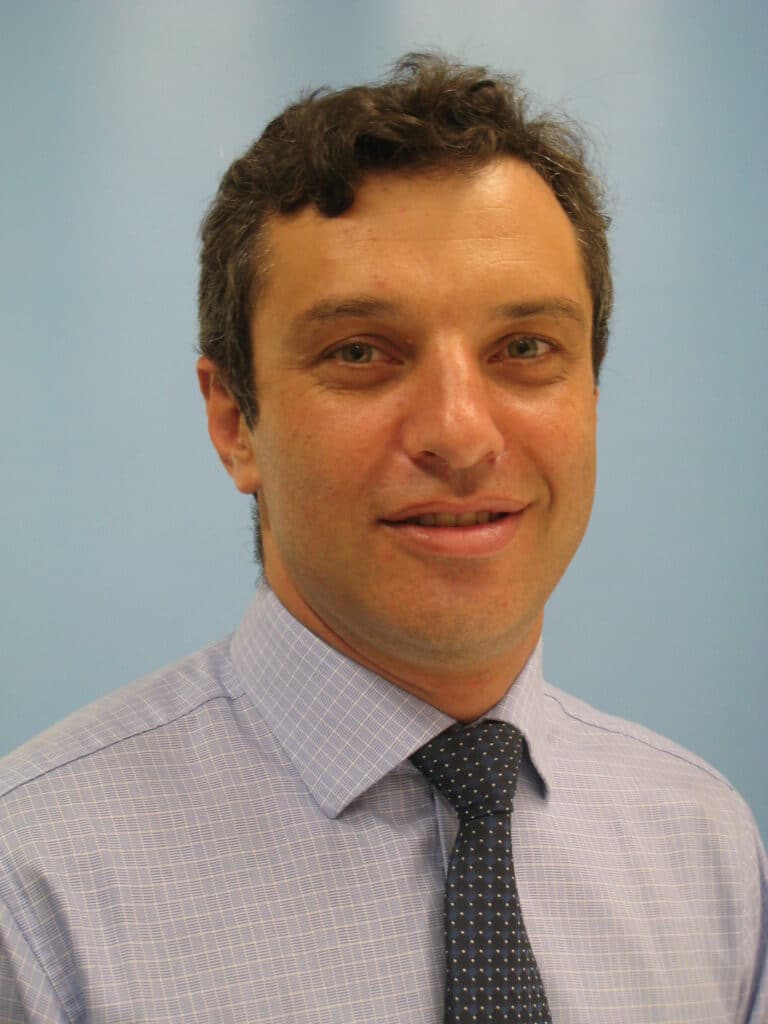
Eugene Kanevsky: Electronic trading is a hugely important part of equity trading in Asia. We continue to see growth in electronic trading at CLSA and in the overall market. This growth will continue as buy-side traders become even more sophisticated users of algorithms.
In addition to expanding algo usage, buy-side traders are increasingly leveraging Transaction Cost Analysis (TCA) to evaluate execution performance, both of their algos and overall. This in turn drives the sell side to provide a more quantitative approach and offer clients execution consultation / quantitative algorithm customization. CLSA sees this an opportunity to differentiate via superior execution.
The buy side’s heightened emphasis on quantitative measurement of execution performance and workflow automation has driven the rapid implementation of algo wheels. The competition on wheels fosters innovation of algorithmic product R&D, and also encourages communication between the buy side and the sell side on understanding individual client’s workflow / benchmarks to strike for execution improvements.
What are the primary pain points for institutional buy-side trading desks?
Joanna Wong: For one, data is fragmented. A lot of buy-side firms share the headache of data accumulation for quantitative measurements. It’s hard to collect meaningful data sets to evaluate a brokers algo performance and consistency with so many broker mouths to feed.
Iliquidity is another pain point. For many small- and mid-cap stocks, it is difficult to compile sufficient datasets and develop consistent trading strategies. Trading in these stocks is full of seemingly random liquidity events and associated price moves, and there is a lack of efficient liquidity-seeking tools in the marketplace.
There are also market microstructure issues pertaining to trading in China. The Stock Connect program facilitated Southbound investors’ access to the Hong Kong market; these traders are familiar with Chinese A-shares trading at a much higher frequency and volatility, and their distinct trading style has created changes to Hong Kong stocks’ order book. Algorithmic Trading for Northbound Connect has grown rapidly; initially we were skeptical that machine learning would be suitable in Mainland China, but with the implementation of CLSA’s real-time Neural Networks, we discovered that Mainland China has the most predictable and actionable short-term trading signals of all Asian markets.
What does AI mean for electronic trading?
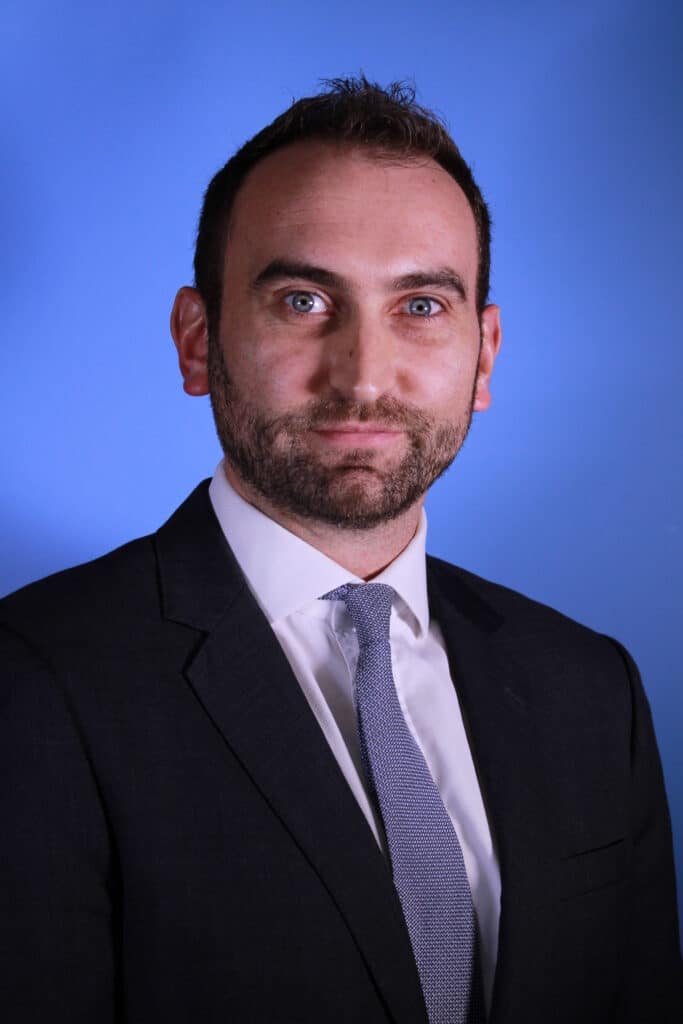
James Redbourn: The integration of artificial intelligence into electronic trading is a game changer. The notion of AI is interpreted broadly with everyone rushing to attach the AI label to their product, but what’s clear is that implementation of AI/machine learning has varied markedly across platforms, and the buy side is faced with algorithmic suites that are fundamentally different. CLSA has been working with proprietary AI-enhanced algorithms to continuously learn, refine and develop these state-of-the-art tools.
But there are limitations. AI at this stage only has meaningful application for short-term prediction. The human trader is still far better at conceptualizing the bigger picture and more adept at making big picture / longer-term decisions.
How specifically is AI applied in electronic trading?
Joanna Wong: We have found AI/Machine Learning to be successful at short-term price-move prediction and refining volume expectations, while statistically quite limited in daily strategy execution. In short, it’s great at forecasting the next move or perhaps the next couple of moves, but not all the moves in a day.
Developing statistically significant short-term predictions was not as difficult as we originally envisaged. The more significant work was developing real-time modules with the ability to dynamically adjust the execution trajectory, while keeping true to the longer view of the trader. The work to develop more complex models, identification of new factors and concepts of game theory to become self-aware of our impact on the market is ongoing.
One key aspect we discovered was that switching from one ‘standard’ algorithm (say, VWAP to inline) can inhibit taking full advantage of the signals generated. Using AI techniques to pick optimal strategy for the rest of the day only yields minor improvements in performance. Switching from one strategy to another makes the assumption of hard boundaries identifying optimal strategy at any point in time. But in reality, there are few hard boundaries, and elements associated with different strategies need to be combined to improve results. We have worked hard to componentize all aspects of trading and use elements of prediction and categorization driven by neural networks to combine the components to suit trading in real time.
What are ‘smart’ algos, and how do they differ from more conventional volume- or schedule-following algos?
James Redbourn: Claiming an algo is smart can be dangerous, as advances in this space are moving at the fastest pace we have ever seen. Algos we once called smart largely operated based on what happened earlier in the day: Is the price better or worse than arrival price, is it better or worse than trailing VWAP. The major shift to what we call smart today operates on the basis of predictions of what’s likely to happen in the future, say the next five minutes of trading. Historic benchmarks still have a place but they generally serve as secondary reference points.
The ADAPTIVE AI framework built into CLSA’s Algorithmic Trading platform leverages Neural Network machine learning technology to generate price, volume, volatility and momentum prediction signals, with real-time market data. These prediction signals give algo strategies a forward-looking view on top of the historical data we reference to create a more comprehensive execution plan. Each algo strategy in our suite can then monetize these prediction signals whilst respecting the strategy’s assigned goal and the trader’s broad execution trajectory expectations.
While the prediction tells the Algos what to target, the how to do that involves a dynamic execution plan that needs continuous revision against real-time market conditions to stay relevant.
Algos we call smart today have forward-looking capabilities, and are able to adjust execution trajectory and order book interaction in real time to deliver more consistent and better performance compared with conventional algos.
What are the unique challenges of trading low-liquidity stocks, and how do algos with smart capabilities help?
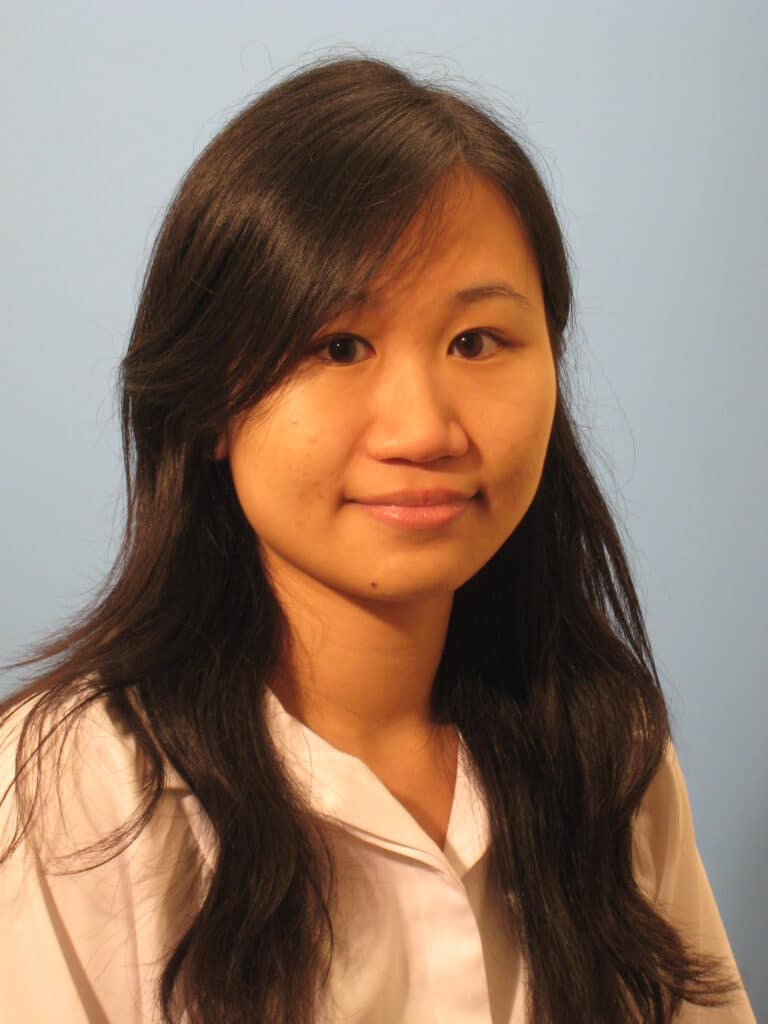
Joanna Wong: Scarce liquidity is always a problem in trading. This is especially the case for small and mid-cap stocks in APAC, which usually have wide spreads and thin order books. Transactions are sporadic with occasional big prints going through ‘randomly’. Research shows for these stocks in Japan, liquidity shock events (defined as over 5% of adv traded in a single print) are observed 2.34x a day on average, and account for 20-40% of the day’s volume. This random but important liquidity appearing makes execution difficult, as outside of the liquidity events, these stocks hardly transact.
Most conventional algos fail to perform well in these stocks. Schedule-based algos can miss liquidity shock events completely; volume-based algos are forced to chase liquidity at unfavorable prices. Thus traders are often forced to manually trade these stocks.
While liquidity shock events are random, it is possible to model how best to trade in this environment. Studies on things like order book replenishment can provide a stable trading trajectory post one of these events, and likewise evaluate options of initiating such an event in conjunction with an effective fair price model.
Opportunistic ADAPTIVE is the latest AI-driven algorithm on the CLSA Electronic Execution Platform. This liquidity-seeking algorithm is built with a machine learning fair price model, moving away from price reference with historical beta of stock with indices. The relationship between stock price, market index and sector index are learned in real time, with more relevance to how the stock is trading today. The algorithm adapts various trading behavior with the guidance from stock clustering on order book information to capture liquidity opportunistically.
How can a sell-side broker differentiate via its suite of trading algorithms?
Eugene Kanevsky: The initial concern voiced with the introduction of AI/Machine learning is that all broker suites would become homogenous. But in reality, nothing could be further from the truth. While many broker algo suites can be rightfully labelled as incorporating AI/Machine learning, the space is broad, and implementations are vastly different.
Continuous investment in R&D is a key to success. We see a lot of competitors set up their algo development as projects, invest time and resources to achieve a specified goal, but then once the relaunch or implementation of the algo suite is complete, they stop the investment.
At CLSA, we started to invest in this space eight years ago, and we launched our first AI algo in 2016. We continue to invest and expand headcount, as we find that each advancement in this space can open up two more areas of research, which tend to be more granular and more specific to a type or particular trading outcome.
Ongoing partnership with clients to tailor-make and fine-tune algorithms for their needs is another differentiator. Our experienced coverage team works closely with clients’ trading and quant teams to help with their execution problems. Supported by our execution quant and algo development team, we proactively monitor clients’ execution performance and propose algo solutions.