By Henry Marriott, Vice President, APAC AES Systematic Coverage, and Nicole Kim, Vice President, APAC AES Sales, Credit Suisse
Problem Solving 101
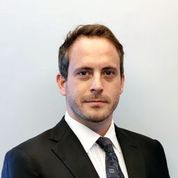
Let’s admit it. We are willing information addicts, reluctant to admit that the constant information overload is simply exhausting. One has to wonder whether this perpetual state of overload is in fact helpful or harmful to our problem solving and decision making processes. So then, what are the steps involved in solving a problem? First and foremost, defining the problem is of course paramount. This is also the most crucial step as it involves asking the right questions to capture the essence of what you want to achieve and to understand your ultimate goal. Then comes the gathering and processing of all of the relevant factors for consideration, before finally sifting through the data to reach a robust answer.
No matter the number of steps involved, it should be stressed again that the most important step lies in asking the right questions. The evaluation of your answer will also heavily depend on getting this first step right. If you don’t even know where you want to go, how will you know whether you’ve reached the right destination?
Importance of Objectives: Lessons from the Plant vs. Meat Diet Debate
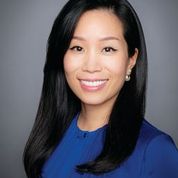
Think about the sheer amount of time, thought, and effort we expend on the problem of what to eat, every day, three times a day (usually). It is no wonder then, that the debate of the plant vs. meat diet has garnered such interest over the years as a topic that speaks to all of us.
The gathering and processing of all the relevant factors to consider for this debate is no easy feat. The complexity behind this seemingly simple topic is compounded by the countless studies and data points that continue to emerge, each defending their merit. From a health perspective, both vegans and meat eaters will argue that their approach is healthier. Some vegans believe meat causes cancer and destroys the planet. But meat eaters will argue that giving up animal foods leads to nutritional deficiencies. We have just looked at the health aspect of the problem, but what if we threw in more elements into the equation such as environmental impact, ethics, religion, cost efficiency, and individual taste? This is where establishing the objective becomes critical. What exactly are you trying to achieve from solving this debate?
We are by no means trying to propose a definitive answer. But we do want to emphasize that depending on what you wish to achieve, that answer may differ hugely. Quick and effective muscle gain could nudge one towards the meat-eater camp while environmental impact could have you with the plant-based dieters. Cost efficiency could swing you yet again to the other camp while taste could do the opposite. Again, the emphasis here is the importance of knowing your objective when solving a problem, as this will serve as a beacon plotting a path for you towards the answer.
Best Execution – Please Explain This to Me!
This same emphasis should also apply to our industry’s quest to solve the problem of Best Execution. We have yet to accurately define this concept beyond beating the VWAP or Arrival benchmarks. The reality is that Best Execution lacks a discrete objective, which is also why we are all over the map in our attempt to solve this problem, and why we also lack a means of definitive evaluation.
We should take pause and wonder, are we asking the right questions? Do we even know what we want out of Best Execution? Going back to the problem-solving sequence, knowing your objective ahead of solving a problem is critical. Thus, viewed in this light, should we not consider aligning Best Execution with each order’s investment objective?
Order Placement Process
The Investment Process, as a whole, is not something that brokers typically concern themselves with, but we are certainly aware that there is a lot going on behind the orders we receive. Indeed, idea generation, research, and establishing investment objectives all come before the placement of an order. However, generally speaking it has become accepted that the elusive solution (if it exists) to Best Execution sits within that Order Placement Process, and this is where the brokers come in.
Not so long ago, the Order Placement Process was relatively straightforward: a PM would deliver an order to a trader with instructions relating to their intention, and the trader would then select an algo to use based on an understanding of the investment objectives. This execution process was tightly coupled with that intention.
Over time, in trying to achieve Best Execution, mechanisms such as the Wheel have been introduced to remove subjectivity and improve efficiency. Now before we receive an order, a lot more is happening. A PM can send an order to a trader as before, or into a Wheel. The trader can send the order to us, or they can place it into a Wheel. Is the ticket we receive from a trader (or Wheel) the same size and price as the original order released by the PM? How is the Wheel configured? Taking it a step further, individual orders are not necessarily generated by a person at all, rather a quantitative model may generate orders autonomously which could then be routed via a Wheel-like process to brokers.
With this extended order placement workflow, brokers can still assess order characteristics (e.g. size, %ADV, mid/large cap) and market conditions, information no doubt valuable for execution. Yet, in our attempts to eliminate inefficiencies, each step and decision introduces separation, and information about the original intent is lost. And if the Investment Objectives do not readily inform the Execution strategy, what does that mean for assessing Execution performance, for example by using TCA?
How much does TCA reflect Execution Performance from the trader/PM’s standpoint?
Typically, TCAs are presented using benchmarks such as Arrival and VWAP – these are fairly straightforward, but in isolation they can only tell us what has happened for the duration of the order.
In the below chart we attempt to add some perspective to these metrics. We combine all of the trades done by each individual trader (for a single client firm) over a specified time period (e.g. a whole quarter) and plot these lines showing the average price move before, during, and after trades are executed.
To reiterate, this chart shows what transpired on average over an entire quarter, so we all but remove random market conditions as a probable cause for the variation in results. So what is going on? The algo did not just behave differently for each trader, but the results clearly differ.
We as brokers can only infer that there are varying underlying strategies precipitating these orders, and then try to draw a path back to that original intention. We could compare algo performance in each scenario in isolation (e.g. by looking at participation rate to try and ascertain whether we are looking at alpha or impact), or we could suggest that there appears to be momentum, or reversion and then make a suggestion. But what if the individual is trading for multiple strategies and/or PMs? What if they just have operational constraints? With context, TCA can be assessed in a more meaningful manner and we are far better placed to provide significantly effective execution advice beyond raw algo performance and optimization.
Align Execution Approach with Investment Objectives
Using TCA or otherwise, we need to expand the dialogue between the buy and sell-sides in order to reconnect the Investment Objectives with the actual execution of the order.
Under the current framework, we often do not know if a ticket we receive is a short-term, stand-alone alpha based trade, or part of a larger, multi-day order to establish a large position with a long time-horizon. If we received two equally sized orders in the same stock at the same time, but in addition we knew those two different objectives were behind each order, our execution approach could differ hugely. Our clients can provide some insight into what they are trying to achieve by giving us a benchmark or assigning an urgency to an order, but this isn’t actually an objective – it is just a proxy for the objective of the investment strategy. As a result, where there is room for some discretion away from a scheduled instruction such as a VWAP basket, brokers have tended towards ‘smart’ spread-capture strategies that are largely intention-agnostic, precisely because we are somewhat unaware of the true intention behind the orders we receive.
In the world of algo trading, the final part of the Investment Process, order execution, is outsourced to brokers with more technology and processes in between than ever before. Brokers can continue to work on a single important factor of Execution, such as optimizing algo logic; however, similar to the Plant vs. Meat example, they are somewhat limited in their ability to contribute to Best Execution without knowledge of the investment objectives. We need to establish the right questions that need to be asked so that trades are put in the context of the overall intention. This is crucial in the mutual goal to execute orders in the most efficient manner, thus truly achieving “Best Execution”.
Intraday Alpha Progression by Trader