Algo wheels have rolled their way into common usage, allowing buy-side desks to select their brokers based on an ever-growing range of factors. But with the influx of data and advanced analytics has come further evolution – bringing with it unique opportunities. Alex Pugh explores how buy-side demands are changing with the times.
The crunching of data has allowed the buy-side to consider and parse exogenous factors, reduce market noise, and observe and quantify changes in flow characteristics to better isolate broker algo performance. In particular, the advancement of peer analysis through clustering groups of orders of similar characteristics and difficulties, ensuring inputs across different brokers are similar, so that clients’ statistical framework can evaluate performance more accurately.
Mehmet Kinak, global head of equity trading at T. Rowe Price, agrees that for the buy-side, algo wheels have aided in both workflow improvement and automation. “In terms of data, the quality of the output is determined by the quality of the input,” he tells Global Trading.
Data, data and more data
Kepler Cheuvreux’s head of electronic distribution, Bobbie Port, also highlights the influx of data, with buy-side desks now having more tools to evaluate and monitor broker performance, leading to more data-driven decisions in their broker selection. “This shift has increased the emphasis on execution quality, transparency, and technology, pushing brokers to adapt and innovate to meet the evolving demands of the buy-side. We are now seeing clients taking our own application programming interface (API) to help them make trading decisions inside their wheels,” Port explains.
Other key considerations from a broker sell-side perspective are the quality of execution, technology, customisation, transparency, compliance and regulation, as well as ‘white glove service’, which pertains to the quality of the relationship between the broker and the buy-side desk.
“Buy-side desks prioritise brokers that consistently provide best execution,” Port says. This involves not just competitive pricing but also high-quality order routing, minimal market impact, and reduced slippage. As data has become more prevalent, buy-side desks use transaction cost analysis (TCA) to assess broker performance quantitatively.
Buy-side desks also look for brokers with robust technology platforms that offer speed, reliability, and scalability. This includes advanced, smart order routers (SOR), and algorithmic trading capabilities. “The ability to provide seamless integration with buy-side systems is also crucial,” Port notes. Additionally, buy-side desks seek tailored solutions, such as bespoke algorithms, customised reporting, or flexibility in execution strategies.
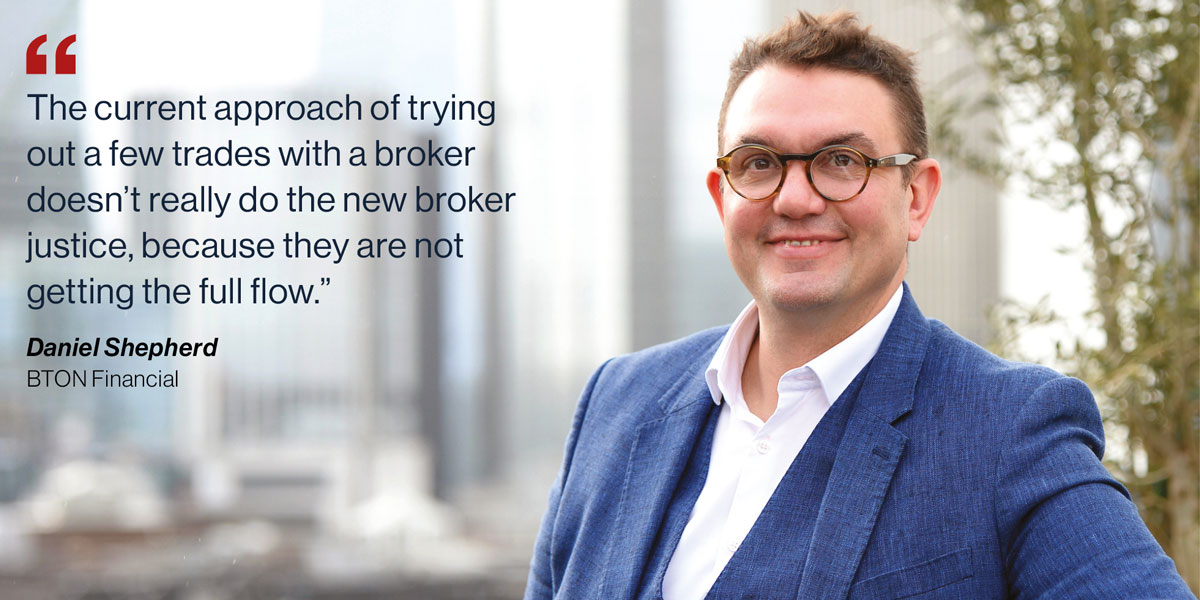
AI improvements
But according to BTON Financial CEO Daniel Shepherd, it can be difficult to assess brokers’ without testing them out. There is no independent third-party platform, a ‘Trustpilot’ for algo wheels, as it were. “That is what we are trying to do, and potentially create some kind of index in the future,” says Shepherd. “The current approach of trying out a few trades with a broker doesn’t really do the new broker justice, because they are not getting the full flow.” Shepherd said BTON is using data to help modernise this relationship and plug the ignorance gap.
BTON’s iWheel technology leverages similar advancements in technology to outperform traditional algowheels. By integrating TCA data from across a wide customer base, the iWheel creates a detailed ‘trading spectrogram’ that analyses performance based on various criteria, including instrument type, percentage of average daily volume, volatility, and benchmarks.
“We use data from TCA gathered from across the customer base. This allows us to get a collective intelligence. Unless you are an extremely large asset manager, your data may not be significantly statistically relevant enough. The bigger the dataset, you can break down performance ratings which allows you to tweak broker selection,” Shepherd adds. “If I’m trading X equity, it should definitely go to this broker because they’ve been incredibly good at those over the past three months.”
Transparency, dynamicity and customisation
“Buy-side desks are more advanced to better assess realised outcomes as it relates to quantifying and comparing different algos and providers,” notes Berenberg’s global head of electronic trading and distribution, Jason Rand. This iterative process is fed back to brokers, which enable them to optimise their strategies leading to better performance and ultimately lower trade implementation costs for end investors.
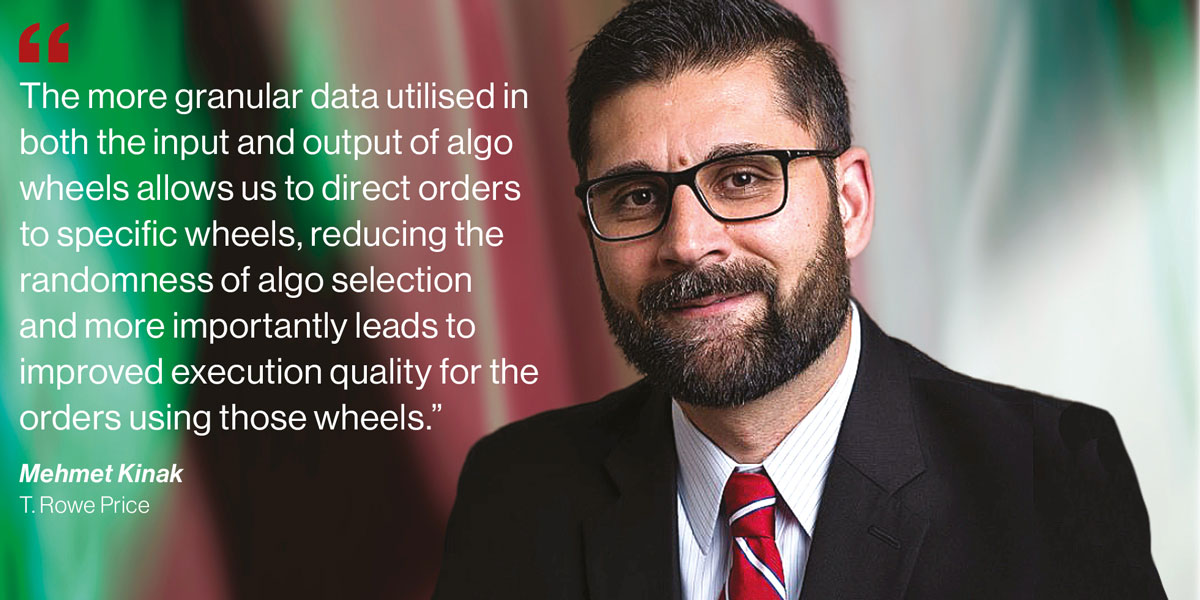
T.Rowe Price’s Kinak notes, “We strive to provide more comprehensive feedback to brokers about their performance in algo wheels, but it’s a delicate balance between incentivising innovation vs. aiming for a prescriptive benchmark.”
“From the viewpoint of a sell-side house, enhanced transparency, driven by improved data analytics, offers several benefits, influencing both client relationships and overall market behaviour,” Kepler’s Port says. Enhanced transparency fosters greater trust with clients – when clients have a clear view of execution processes, pricing, and other trading details, they are more likely to trust their brokers. “This trust is a fundamental pillar to building long-term client relationships,” adds Port. “With increased transparency, brokers are under pressure to demonstrate the value they provide to clients in terms of execution quality, efficiency, and cost-effectiveness.”
Therefore, brokers are more proactive in sharing execution data and performance metrics with clients to showcase their capabilities and differentiate themselves from competitors.
Enhanced transparency also helps ensure compliance with regulatory requirements. With stricter regulations like MiFID II, providing clear and accurate data on trades and execution processes is essential.
Working together
Berenberg’s Rand says the development and integration of algo wheels by the buy-side community has created noticeably greater efficiencies for trading desks. The allocation of flow within different wheel buckets can be based on a variety of factors including benchmark performance, liquidity capture, economics, and many other qualitative and quantitative considerations. “The inherent limitations of algo wheels originate due to their lack of dynamicity in employing broad data sets and real-time market signals to assess the best type of algo/broker/strategy that yields the optimal outcome of the client objective at a given point in time throughout the trading day,” Rand points out.
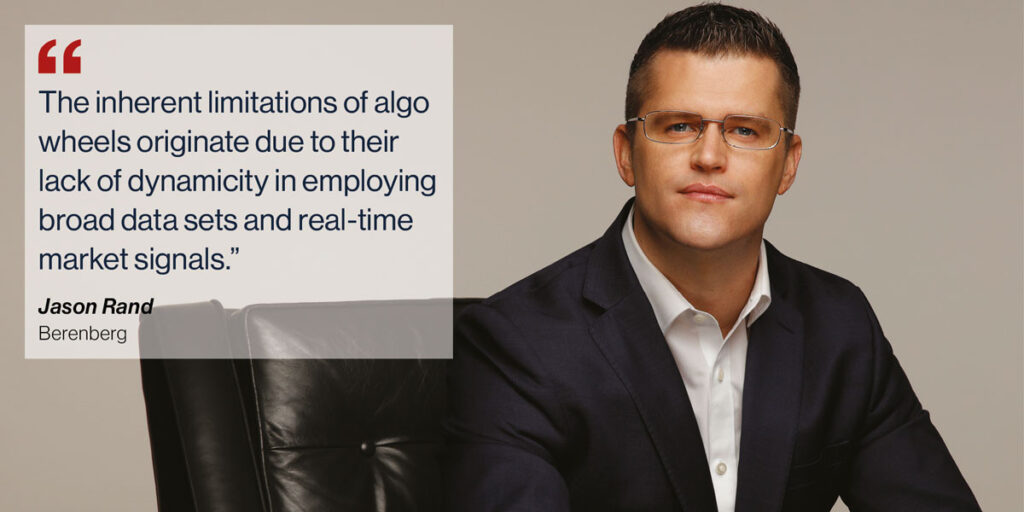
Kepler’s Port thinks algo wheels could benefit from dynamic adjustments based on real-time performance metrics. For example, if an algorithm consistently underperforms in certain conditions, the wheel could automatically adjust to reduce the frequency of selection for that algorithm. “This dynamic approach would enhance the adaptability of the algo wheel, ensuring that order flow is directed toward algorithms with better performance,” Port adds.
And by allowing customisation of parameters within the algo wheel, sell-side firms can better cater to specific client needs and trading strategies. This could involve setting constraints or preferences for specific algorithms based on market conditions, asset classes, or other factors. “Customisable parameters provide greater flexibility in the selection process, enabling brokers to align the wheel with their clients’ end goals,” Port noted.
Algo wheels could also be improved by integrating feedback mechanisms that collect data on the effectiveness of the selection process. This feedback could come from internal performance analysis or direct client input. “Regarding randomisation in algo wheel selection, some degree of randomisation is typically introduced to ensure unbiased distribution of orders among multiple algorithms,” says Port. “A balance between randomisation and logic-based adjustments is key to creating a well-functioning algo wheel. To improve algo wheels, sell-side firms can focus on enhancing algorithm selection logic, introducing dynamic adjustments, offering customisable parameters, and integrating feedback mechanisms.”
Kinak says that, initially, orders for algo wheels were identified by using a single metric like % ADV. “More recently, we’ve been able to categorize the orders themselves in terms of size, alpha and difficulty and coupled with a better understanding of each algos’ behaviour, our comparison of results has become more accurate and reliable.
“The more granular data utilised in both the input and output of algo wheels allows us to direct orders to specific wheels, reducing the randomness of algo selection and more importantly leads to improved execution quality for the orders using those wheels,” Kinak adds.
AI, algos and markets
So where are we now? Recent developments around scaling close algorithms, get-done strategies based on impact costs and timing risks, dynamic arrival based and liquidity seeking algos, and direct-to-capital optionality have all helped to move the needle. Another key main improvement has been to improve short term price and volume prediction models, to lessen signalling and adverse selection amid declining volumes.
But could investment into algorithms have now peaked? Some feel that the economics and commercial opportunities are prohibitive to new future participants successfully entering the space, thereby limiting the main benefits of market competition and innovation.
And while AI might seem to be the future, others are less sure. “While there are some participants claiming to leverage AI, the integration of such advancements into trading algorithms, which essentially make decisions through non-deterministic processes, creates a significant barrier to mass adoption,” notes Rand.
If an AI-driven algo makes a decision based on its interpretation of interconnected events, and that decision results in a wide standard deviation of performance, the challenge remains for both trading desks and providers to explain that in a digestible format to compliance teams. The inherent black box nature of some machine learning algorithms makes traceability and replicability of their signals in a controlled environment challenging.
“It’s equally important to remind ourselves that markets are ever-evolving and that there is no guarantee that an AI-driven trading algorithm that was fitted, trained, and tested at a point in time in the past will deliver similar results in the future,” Rand warns.
©Markets Media Europe 2024 TOP OF PAGE