When using algo wheels, traders will leverage transaction cost analysis (TCA) to determine which broker is best, but data barriers often present a challenge. Nonetheless, integrating analytics and data into broker selection allows algo wheels to continuously improve.
According to a report from FlexTrade, Enhance Institutional Trading Performance: Leveraging AlgoWheels and Advanced Cost Models, many of their clients must collate disparate data sources and sift through mountains of data to glean insights. Collecting, normalising, and analysing data, as well as achieving the sample size necessary to derive value from trading experiments, are common problems, the report suggests. Additionally, in terms of data, the quality of the output is often determined by the quality of the input.
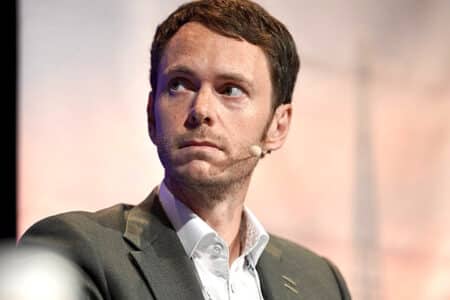
FlexTrade managing director, EMEA, Andy Mahoney, told GT that modern algo wheels have moved beyond the original construct of simple, pre-configured automating rules.
“Today, a modern algo wheel is designed to provide maximum flexibility in constructing automation for testing and rules, with simple drag-and-drop tools that can be used without IT assistance.
“Taking things a step further, traders can incorporate TCA data and in-flight analytics to create a real-time feedback loop that automatically prioritises better performing destinations and liquidity sources, while decreasing allocation to those missing the target. Traders can use multiple performance metrics to refine an algo wheel, ensuring continuous trading improvement based on the order profile rather than being hardcoded to one dimension,” Mahoney added.
Institutions also struggle with transaction cost analysis, the report noted, as well as market noise and sample size. Volatility, market events, and trading activity all cloud analysis of brokers and strategies. And in order to draw meaningful conclusions from algo wheel or TCA results, there needs to be enough historical data.
Parameter normalisation also creates headaches, as firms attempt to stitch and map data together from different brokers. Additionally, industry sectors can have large intraday moves that add an additional hurdle for comparison.
FlexTrade posits that transaction cost models, which remove market noise and normalise data sets, allow institutions to properly analyse broker performance. As an input to the algo wheel, the normalised data is used to draw comparisons to discover the optimal mix of brokers, algorithms, and strategies. “This is further improved as desks adopt experiments as part of the process, which helps them gain a competitive edge,” the report noted.
©Markets Media Europe 2024