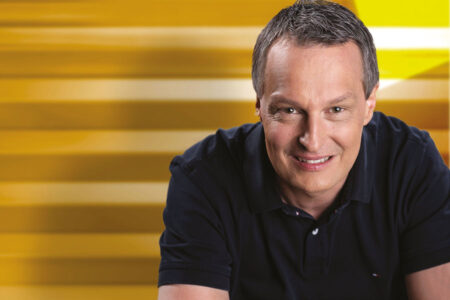
AI and machine learning is helping banks strip manual work out of reconciliations processing, says Andreas Burner, CTO, SmartStream, and with customers realising the additional business benefits this technology brings, more and more are now trying it out for themselves.
The last eighteen months have witnessed a surge of public interest in, and acceptance of new technologies. Amongst financial institutions there is also an increasing willingness to embrace machine learning (ML), large language models (LLMs), and other artificial intelligence (AI) technologies that help them to optimise their business operations – in the November 2023 IBM Global AI Adoption Index (Enterprise Report), half of financial services respondents reported that their enterprise had actively deployed AI.
Financial services firms are employing AI in a variety of areas, from fraud detection and credit scoring, through to customer service chatbots. Amongst major institutions an appetite for AI is especially apparent, and some have multiple use cases in production. JP Morgan Chase CEO, Jamie Dimon, commented in an annual letter to shareholders that the bank had more than 2,000 AI/ML experts and data scientists, and over 400 AI use cases in production.
Another sphere where AI offers exciting scope is the back office. Burdensome overheads, mounting transaction volumes, and an ever-growing public demand for instant payments are exerting increasing pressure on banks, and some are already investigating emerging AI-based technologies in the search for new operational efficiencies and a more cost-effective path forward.
Understandably, fears around AI still linger, however, driven by concerns relating to security, privacy, system failure, explainability, legal responsibility for decisions made by AI, ethical risks and so on. Yet these worries should not hold firms back from experiencing the benefits advanced AI-based technologies can provide. To overcome this hesitation, financial institutions need to see viable use cases where AI-based solutions have been rigorously tested and are proven to work and add value.
SmartStream’s innovations team, which is staffed by a team of experienced data scientists, mathematicians and technologists, focuses solely on developing AI and ML-based solutions aimed at solving the business challenges faced by its customers. Harnessing AI to eliminate time-consuming manual effort from reconciliations processing is an area of particular interest to the team.
Once a bank has run its main reconciliations system, a pool of unmatched transactions is generally left over. Human reconcilers must then marry up the data, which takes time, skill, and effort, driving up overheads. In response, the innovations team has developed a solution called Affinity. This observational AI tool is deployed as a module for SmartStream’s market-leading TLM Reconciliations Premium technology, which is designed to maximise the value customers derive from their existing investments.
Affinity operates by observing how experienced human reconcilers link transactions together. Having watched how these links are successfully created, it then applies this learnt logic to fresh information and presents the best possible correlations between outstanding records. Human users can either accept or override Affinity’s suggestions, allowing oversight and control. Each prediction is accompanied by an explanation showing the specific data attributes on which the AI has based its decision, providing transparency and explainability for compliance purposes.
Importantly, Affinity can handle great complexity and large quantities of data, allowing it to detect relationships beyond those discoverable by the human eye – meaning it can pick up anomalies and subtleties which might otherwise go unnoticed.
Affinity delivers genuine, real-world benefits. A recent study, carried out by a large US bank and SmartStream data scientists, illustrates how Affinity, which uses advanced machine learning technology, can cut the amount of costly effort firms spend on manual intervention.
The bank uses SmartStream’s TLM Reconciliations Premium to process reconciliations. The rules-based solution was configured to automatically match as many records as possible, but a group of unresolved transactions typically remained after the system had run. Human intervention was therefore necessary, and the bank wanted to see how effectively AI-based technology could tackle these outstanding records.
During the first leg of the study, which was conducted against a securities reconciliation, experts from our own innovations team were given several weeks of historical data on which to train Affinity. The AI was asked to learn patterns and trends from the manually matched data only. Affinity was then provided with fresh, unseen data, on a daily basis, and simulations carried out to see how accurately it drew together the new records.
Taking a snapshot over an eleven-day period, 52.7% of transactions were matched by Affinity from the set of records that a human user would have investigated. Some 50% of human effort was required for this reconciliation when using Affinity, meaning a potential doubling of FTE productivity. Turning to time savings, some 42 hours of actual FTE time were necessary to draw together 15,000 records. In comparison, Affinity took under 3 hours to correlate the same records.
A further example underscores Affinity’s usefulness. A customer, based in the Middle East, uses TLM Reconciliations Premium to carry out reconciliations processing activities. Auto match rates were very high (95%), but the bank wanted to further reduce manual touch points and review costs.
A similar example was run against a cash reconciliation, with Affinity trained on historical data, and a simulation then carried out to see how effectively the AI performed against new, previously unseen data. We focused on representative samples from a group of accounts chosen by the client, some 13,407 records, and Affinity achieved a 43% time saving (i.e. compared to the time human reconcilers required to investigate the same transactions).
As these real use cases show, Affinity creates time-saving and productivity gains, and therefore facilitates a reduction in overheads. It should be noted, however, that time-savings may vary, and are especially dependent on data quality.
Perhaps even more importantly, Affinity retains knowledge within a company: when resources leave or retire, their knowledge does not depart with them, but is ‘institutionalised’ into the solution, promoting operational sustainability. It therefore plays an important role in reducing the significant burden companies are placed under by staff attrition. Additionally, by reducing repetitive work, Affinity frees staff up and allows them to be redirected to more productive tasks.
In conclusion, while banks are already making use of AI and machine learning, for example, in fraud detection and risk management, AI-based technologies also offer plenty of scope to inject efficiency into back-office operations. With proven, operational technology available, banks can have greater confidence about using AI to remove cumbersome manual intervention from their reconciliations activities – indeed, they rapidly need to become confident adopters, if they are to avoid being left behind by more agile, technologically advanced competitors.
©Markets Media Europe 2024