Large language models such as ChatGPT have become a global sensation since demonstrating an ability to conduct convincing conversations, pass exams and produce text. Meanwhile, the European Commission is consulting on new AI regulations. But how useful are these AI systems for traders?
Four researchers – Han Ding, Junhao Wang and Yinheng Li at Columbia University and Hang Chen of New York University – explore this question in a review paper published on the Arxiv Trading & Market Microstructure forum, where they examine 27 papers on LLM-based trading. In addition to their academic affiliations, Ding works as a data scientist for Amazon, Wang for Curemetrix LLC, Li is a scientist at Microsoft and Chen is an engineer at Snap Inc.
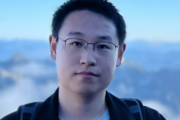
According to the authors, LLMs can be deployed as trading agents, directly generating and executing trading decisions, or as ‘alpha miners’, proposing and testing investment ideas which can be later implemented by humans. Backtesting shows average trading performance of 15-30% over benchmarks, although this is not the same as a real-world test.
The simplest type are ‘news-driven’ LLMs, which are fed corporate and macroeconomic news updates in order to directly predict stock price movements, similar to earlier-generation machine learning AIs. Then there are ‘reflection-driven’ LLMs, where news feeds and trading outcomes are summarised as memories than can be drawn upon when new information arrives, mimicking the human ability to reflect upon experience.
Debate-driven LLMs consist of multiple agents, each with a differing personality, that debate each other about investing strategies, which the research shows improves their trading performance. Reinforcement learning-driven LLMs are shown historical outcomes from financial markets to refine their predictions.
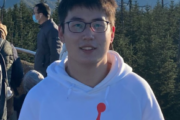
The alpha-mining LLMs start with a ChatGPT-style conversation, where a trader prompts the LLM to generate a trading idea in the form of a script. Then another LLM is brought in as a judge providing feedback to refine the script, in the form of code which is tested in the real world, with the feedback used to improve both agents.
As regards the choice of LLM, OpenAI is the platform of choice in the studies surveyed by the authors. Data is a challenge, though, because LLMs are (as their name suggests) designed to handle text inputs rather than numerical stock price data. This works fine for financial reports such as 10-Q filings or analyst research. But for stock price histories, the only way forward is to convert numbers into text strings, which the LLMs process like a conversation.
The EU Commission’s July consultation paper has a section on securities markets that could cover a number of situations using LLM-based trading algorithms. Referring to generative AI as ‘general purpose AI’, the Commission asks industry respondents whether systems that act similarly could lead to herding effects, or whether trading algorithms could collude to manipulate markets and squeeze liquidity.
The fact that OpenAI’s platform dominates current LLM trading efforts, suggests that herding should be a concern, given that the trading agents ultimately derive their insights from the same corpus of OpenAI training data. And one can imagine debate-driven LLMs – if not properly supervised – privately agreeing to breach securities laws in order to boost profits.
In a question about ‘robo-advice’ the Commission frets about LLM hallucinations harming consumers. Having read Ding, Li, Wang and Chen’s review paper, it’s an amusing pastime to think about the market scenarios that could result if LLM trading agents become widely adopted.
©Markets Media Europe 2024